Real-world evidence (RWE) is a critical tool for understanding how medicines perform in diverse patient populations and real-world settings. However, the sheer volume and complexity of RWE data pose challenges for traditional analysis methods.
Artificial intelligence (AI) is revolutionizing the pharmaceutical industry and having a major impact on value and access (V&A). By harnessing AI to extract RWE insights, developers can efficiently demonstrate the value of medicines, broaden patient access and strategically navigate the complexities of market access.
In this blog, Envision Pharma Group leaders Suki Kandola, Global Head of Commercial Strategy, Value and Access and Yahya Anvar, General Manager and Head of Okra.ai explore the current challenges in leveraging RWE in V&A and how AI can extract insights to generate a comprehensive and robust evidence base that feeds into V&A strategies.
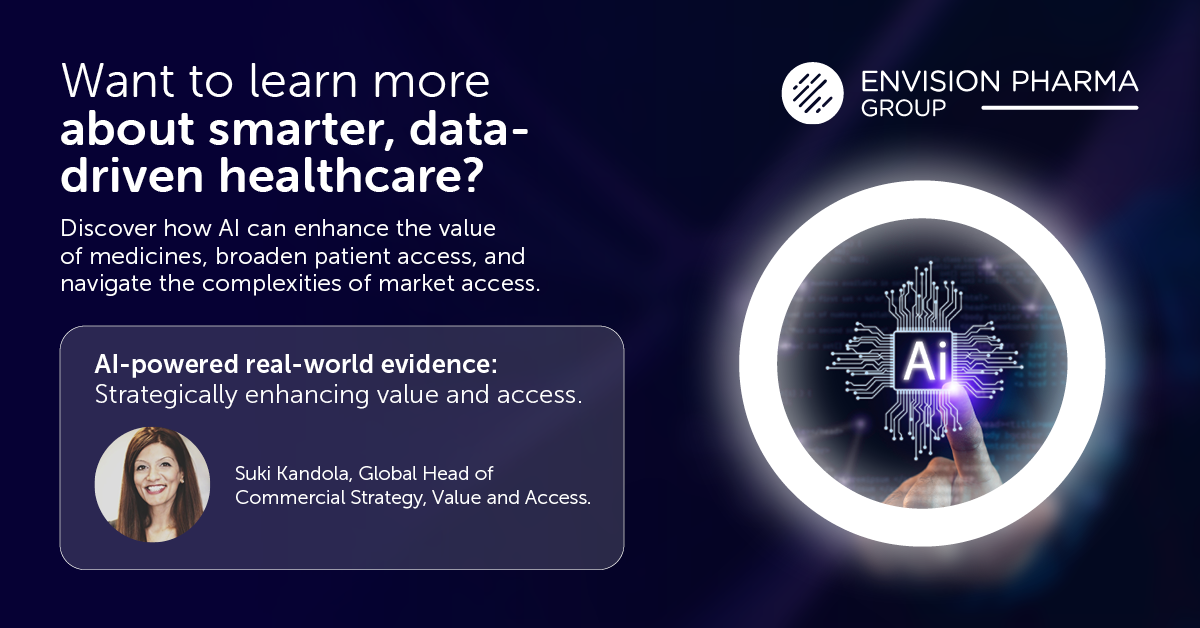
Informing healthcare decisions with real-world insights
Unlike traditional randomized controlled trials (RCTs), RWE captures data from diverse sources, such as electronic health records, insurance claims, patient registries and wearable devices. This wealth of information provides a more comprehensive picture of how treatments perform in real-world settings, taking into account a wider range of patients, comorbidities and treatment patterns.
The significance of RWE lies in its ability to complement and enhance the findings of RCTs. While RCTs remain the gold standard for establishing efficacy and safety, their controlled environments often mean results are not reflective of real-world populations. By providing insights into long-term outcomes, comparative effectiveness and the impact of treatments on various patient subgroups, RWE bridges the gap between RCT data and real-world populations. This information is invaluable for healthcare decision-makers, including payers, regulators and clinicians, who need to make informed choices about coverage, reimbursement and treatment guidelines.
Extracting RWE insights with AI
Natural language processing (NLP) has proven particularly useful in RWE analysis. These algorithms can extract relevant information from unstructured text data, such as clinical notes and patient narratives, which often contain valuable insights about treatment outcomes, adverse events and patient experiences. By integrating AI and NLP, companies can help overcome RWE challenges to demonstrate value and develop successful access strategies.
Data quality, availability and structuring
Real-world data is often unstructured and can be biased due to varying collection methods, underrepresentation of certain populations, or missing data. Accessing and integrating diverse real-world data sources, such as electronic health records, claims data, and patient registries, can be challenging due to privacy regulations, data security concerns, and interoperability issues.
NLP and machine learning techniques can help solve these challenges by identifying and quantifying biases in unstructured textual data, enabling data augmentation and bias mitigation algorithms to create more representative datasets. Additionally, NLP can extract valuable information from unstructured sources, standardize terminology across diverse datasets, and de-identify sensitive data, improving data accessibility while maintaining patient privacy.
Regulatory and health technology assessment (HTA) approvals
Concerns about data quality and relevance, including heterogeneity, missing data, and confounding factors, can limit the use of RWE in HTA approvals. Study design and methodology issues like lack of randomization, selection bias, and complex statistical analysis can also impact the reliability of RWE findings.
AI approaches can identify missing data and help with data structuring and quality improvements. This provides a better understanding of the target population and establishes a mechanism to supplement RCTs and other observational studies in line with global HTA requirements.
Targeted evidence generation and value propositions
Tailoring value propositions using RWE is challenging due to the complexity and heterogeneity of real-world data. Identifying specific patient cohorts requires advanced analytics and data integration capabilities and ensuring data privacy and maintaining ethical considerations while targeting specific populations can be complex. Balancing the need for granular insights with the risk of over-segmentation and small sample sizes is crucial for generating meaningful and reliable evidence. Translating RWE into actionable insights requires effective communication and collaboration between different stakeholders.
AI and NLP can be used to identify specific patient cohorts within RWE, generating targeted evidence that directly addresses concerns preventing approval in a particular region/country or for a specific subpopulation. By identifying unmet medical needs or areas of clinical practice that are not well understood and estimating the potential burden on caregivers and society, AI can also help demonstrate how new treatments are likely to alleviate this societal burden.
Additionally, analyzing individual patient data from RWE can identify subgroups that might benefit most from a product. This information can be used to tailor marketing and educational efforts towards specific patient populations, enhancing the product's relevance/value and appeal to healthcare providers.
Demonstrating value and informing clinical practice
Ultimately, AI-driven RWE can enhance clinical practice and patient care. By providing information on product efficiencies, optimal treatment plans, and insights into safety, efficacy, and cost-effectiveness in real-world settings, RWE can inform clinical practice and reimbursement guidelines. The ability to analyze product effectiveness and capture patient-reported outcomes (PROs) enables a deeper understanding of patient experiences, fostering shared decision-making between patients and healthcare providers. This comprehensive approach ultimately contributes to improved treatment strategies and better patient outcomes.
Although AI-driven RWE evidence approaches can revolutionize V&A strategies, harnessing the full power of AI can be challenging for developers. The AI landscape is progressing rapidly. Models are complex and constantly evolving, which means they require technical expertise and resources to comprehensively understand and harness them. Developers also need to consider data quality and any biases that can arise when analyzing with AI tools. These challenges are linked to the ethical use of AI and ensuring that tools meet the relevant regulatory standards.
Leveraging AI-powered RWE to drive value and access
To fully harness the potential of AI-powered RWE, drug developers can collaborate with strategic partners who possess the expertise and resources to navigate the complex AI landscape. Partnering with experts in both AI and RWE enables developers to overcome challenges and implement a comprehensive V&A strategy.
The convergence of AI and RWE is revolutionizing the way the value of medical interventions is demonstrated, leading to more effective V&A strategies and enhanced patient access. Partners with deep knowledge and experience in V&A strategies and specialist AI platforms can help drug developers navigate the complexities of RWE and maximize the impact of their evidence-generation efforts. As AI continues to advance, its potential to transform the healthcare landscape will only grow, ultimately leading to better patient care and improved health outcomes.
In the next part of this blog series, we will explore OKRA.ai’s RWE brain. Discover how Envision Pharma Group can support your AI-driven RWE initiatives to demonstrate value and maximize patient access: https://www.envisionpharmagroup.com/solutions/market-access